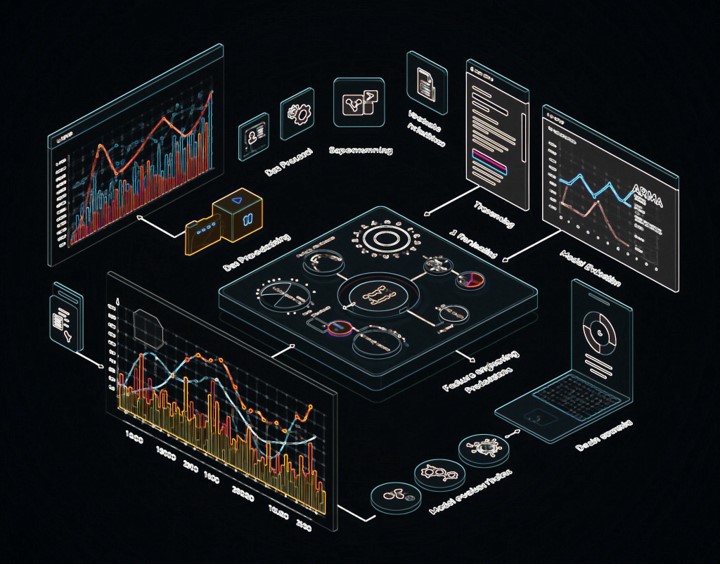
Automatic forecasting for non-Gaussian time series
Automatic forecasting algorithms are crucial tools for many modern-day business operations. Yet nearly all of the available algorithms, and their associated software packages, assume that the series can be modelled with Gaussian errors. This makes it hard for end-users to employ these models when they routinely deal with non-Gaussian series, such as counts of sales, compositional data that must sum to 1, or time series that present as proportions. This project will develop extremely efficient and adaptable forecasting algorithms that can work with a huge variety of real-world time series, filling a crucial gap in the available software landscape for industry-level forecasting purposes.
The student will be based at
The University of Queensland within the School of Veterinary Science, primarily supervised by
Dr. Nicholas Clark. The candidate will work with a diverse group of international researchers. Additional support will be given by partners at the Ecological Forecasting Initiative and the Spatial Epidemiology Laboratory, including assistance in computer-based data analysis, model building and scientific communication. The selected student will have the opportunity to work with all partners on this project but will be based at UQ.
This project will help develop the candidate’s skills in critical thinking, project management, data management and analysis, writing and communication. Expected applications of the project are incredibly diverse, meaning the student will be well prepared for a future career in research or with government and non-government land management, biosecurity or conservation agencies.